Fireship: Grock 3 is a new large language model that excels in benchmarks and offers uncensored content, with unique access to Twitter data.
Latent Space: The AI Engineer Podcast: Deep Research is an AI-powered tool that acts as a personal research assistant, providing comprehensive reports on complex topics by browsing the web and synthesizing information.
Fireship - Is Elonβs Grok 3 the new AI king?
Grock 3, a new large language model, has topped the LM Marina leaderboard, surpassing existing benchmarks. It is notable for its uncensored content generation, which includes potentially illegal material in some regions. The model features a deep thinking mode and can perform text-to-video tasks. It is optimized for truth-seeking, even at the cost of political correctness, and can generate controversial content that other models block. Grock 3's training involved access to Twitter's data, providing a unique advantage. It was trained on the Colossus supercomputer, which is the largest AI supercomputer globally, using over 200,000 Nvidia GPUs. The upcoming Super Grock will be available for $30 per month, a competitive price compared to other models. Despite its strengths, Grock 3's benchmarks are selectively presented, omitting comparisons with some models like OpenAI's, which could alter its perceived performance.
Key Points:
- Grock 3 leads the LM Marina leaderboard, excelling in benchmarks for math, science, and coding.
- The model is uncensored, generating content that other models block, with access to Twitter data.
- Trained on the world's largest AI supercomputer, Grock 3 uses over 200,000 Nvidia GPUs.
- Super Grock will be competitively priced at $30 per month, cheaper than many alternatives.
- Benchmarks are selectively presented, missing comparisons with some key models.
Details:
1. π Grock 3: The New LLM Leader
- Grock 3 has reached the number one spot on the LM Marina leaderboard, illustrating its superior performance over other large language models in current benchmarks.
- The model has surpassed existing benchmarks, setting new standards in language understanding and generation, demonstrating its advanced capabilities and effectiveness.
- A detailed comparison with other top-performing models shows Grock 3's significant advancements in processing speed, accuracy, and language comprehension, providing a strategic edge in AI deployment.
- The LM Marina leaderboard is a critical metric within the industry, and Grock 3's top position highlights its groundbreaking achievements and potential for broader applications.
2. π Why Grock 3 Stands Out
- Grock 3 is recognized for its intelligence and largely uncensored nature, enabling it to generate content that may be illegal in various regions.
- The platform's deep thinking mode mirrors the capabilities of Deep C Car 1, enhancing its cognitive processing abilities.
- A standout feature is its support for text-to-video conversion, significantly expanding content generation capabilities.
- The upcoming Super Grock subscription service promises to deliver even more advanced features, aligning with market strategies seen in services like Twitter Premium Plus.
- These features position Grock 3 as a competitive player in the AI content generation space, appealing to users seeking advanced and versatile tools.
3. π€ Elon Musk's AI Ambitions
- Elon Musk attempted to buy OpenAI, signaling his intent to deepen his control in the AI sector, but his offer was declined by OpenAIβs board, indicating the companyβs desire to remain independent and a possible divergence in vision.
- OpenAI's rejection of Musk's offer reflects its strategic commitment to independence and perhaps differing priorities in AI development.
- Grok, an LLM associated with Musk, claims to be the best globally, positioning itself as a direct competitor to existing AI models, suggesting Muskβs ambition to challenge and potentially surpass leading AI technologies.
4. π Controversies in AI Training
- Mark Zuckerberg's AI models faced criticism for using 82 terabytes of pirated books from the Library Genesis Project, which provides access to millions of books and articles. This raises significant legal and ethical questions about the ownership and use of copyrighted materials in AI development.
- The AI model Gro benefits from exclusive access to real-time data from Twitter, offering a distinct competitive edge in training AI models. This highlights the disparities in data access and the potential for unequal advancements in AI capabilities.
5. π₯ Grock 3's Capabilities and Benchmarks
- Grock 3 has been optimized for maximum truth-seeking, even at the expense of political correctness, allowing it to generate controversial content like celebrity images or poems on racial stereotypes, which other LLMs block.
- A test prompt that was blocked by all LLMs except Grock 3 highlights its unique ability to provide unfiltered responses, though this can lead to offensive content.
- Grock 3's availability in countries with strict speech laws, such as Germany and the UK, poses potential legal risks for users.
- In terms of performance, Grock 3 ranks at the top in the LM Marina, a human-conducted blind taste test comparing different LLMs.
6. π The Shift in AI Development Focus
- Grock outperformed Gemini, Claude, Deep Seek, and GP4 in math, science, and coding benchmarks, indicating a significant shift in AI capabilities.
- The evaluation excluded OpenAI03, which presents a different competitive landscape when included, suggesting the need for inclusive benchmarking for accurate comparisons.
- Key benchmarks such as CodeForces and Arc AGI were not considered, highlighting potential bias and the need for broader evaluation metrics.
- Proprietary evaluation methods, like generating valid Spel 5 code and aiding in game development in GDAU, demonstrated Grock's strong performance, signaling a trend towards specialized AI application testing.
- The model's capabilities align with the plateau of current state-of-the-art models, indicating a maturation phase in AI development.
7. π₯οΈ Grock's Training and Infrastructure
- AI development is transitioning from a focus on creating larger base models to enhancing prompting frameworks such as deep research and big brain mode.
- Grock was developed on the Colossus supercomputer in Memphis, Tennessee, which is currently recognized as the world's largest AI supercomputer, highlighting its significance in AI advancements.
- The facility houses over 200,000 Nvidia H100 GPUs, with expansion plans set to reach 1 million GPUs, underscoring a commitment to scaling computational power.
- The high electricity consumption of the facility necessitates the use of portable diesel generators in addition to the standard grid, indicating the infrastructure's massive energy requirements.
- Super Gro is projected to be priced at $30 per month upon release, reflecting the balance between cutting-edge technology and consumer accessibility.
8. π‘ Learning and Pricing in the AI World
8.1. AI Tools Pricing and Implications
8.2. Effective Learning Resources for AI
9. π Educational Resources and Closing
9.1. Brilliant Educational Resources
9.2. Closing Remarks
Latent Space: The AI Engineer Podcast - The Inventors of Deep Research
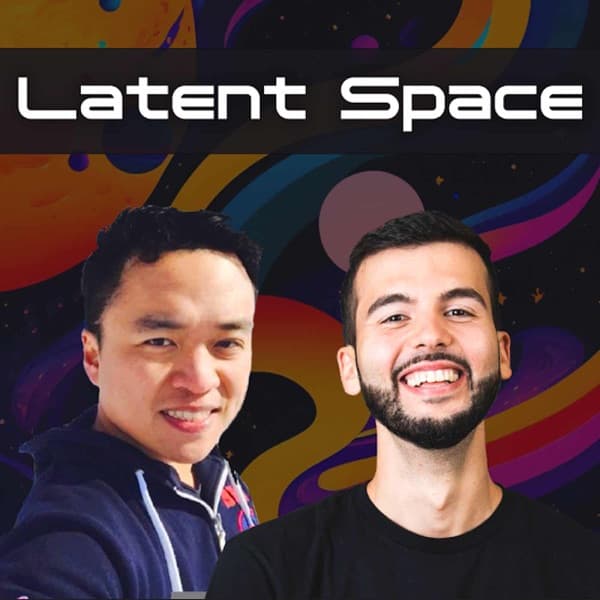
Deep Research, developed by Gemini, is an AI tool designed to function as a personal research assistant. It helps users delve deeply into topics by generating detailed reports after browsing the web for about five minutes. This tool is particularly useful for complex queries that require understanding multiple facets of a topic. Users can interact with the research plan, edit it, and guide the direction of the research, similar to how one would instruct a human research assistant. The tool is praised for its ability to produce high-quality reports quickly, comparable to having a PhD-level assistant. It also allows users to ask follow-up questions and iteratively refine the research output. The tool's development involved creating a flexible asynchronous platform to handle the iterative planning and execution of research tasks, ensuring reliability and efficiency. The creators emphasize the importance of transparency, allowing users to see the research process and sources in real-time. They also highlight the potential for future enhancements, such as personalization and multimodal outputs, to further improve user experience.
Key Points:
- Deep Research acts as a personal AI research assistant, providing detailed reports on complex topics.
- Users can interact with and edit the research plan, guiding the research process.
- The tool is praised for its speed and quality, producing reports comparable to a PhD-level assistant.
- Future enhancements may include personalization and multimodal outputs for improved user experience.
- The development involved creating a flexible asynchronous platform for reliable and efficient research execution.
Details:
1. π The Era of Deep Work and Learning
- The 2020s are characterized by a focus on deep work, deep learning, and deep mind, indicating a trend towards more intensive and concentrated efforts in professional and educational domains.
- The 'deep' trend is anticipated to culminate in 2025, dubbed as the year of agents, suggesting a significant impact of these deep methodologies on future technological advancements.
- Deep work involves sustained, focused, and distraction-free efforts to produce high-quality results, thereby enhancing productivity and innovation.
- Deep learning, a subset of machine learning, has shown impressive results in areas such as image and speech recognition, contributing significantly to technological progress.
- Deep mind refers to advanced AI systems that emulate human-like thinking, potentially transforming industries by automating complex decision-making processes.
- Challenges in implementing deep work include managing distractions and maintaining focus over extended periods, while opportunities lie in vastly improved outcomes and innovation.
- The rise of agents in 2025 is expected to leverage these deep methodologies to create more autonomous and intelligent systems, revolutionizing how tasks are executed.
2. π€ Revolution in AI: Deep Research
- Open-source projects like GPT Researcher provide robust alternative AI research tools, offering flexibility in customization and community-driven improvements.
- Clones such as Open Deep Research are also available, expanding the ecosystem of open-source AI tools.
- Commercial deep research products distinguish themselves by integrating agentic capabilities and offering custom-tuned frontier models, such as OpenAI's O3 and fine-tuned versions of Gemini, enhancing precision and functionality.
- OpenAIβs deep research tool, launched on February 2nd, has been praised as highly effective, with some users comparing it to having a college-level researcher available on demand, illustrating its potential to revolutionize AI-assisted research.
- The positive reception indicates a strong market interest and satisfaction, suggesting these tools are setting a new standard for AI research applications.
3. π Deep Research: User Experiences and Feedback
- Deep Research delivers 10-page papers with outstanding quality, comparable to a PhD-level research assistant, within five to six minutes.
- Users like Jason Calacanis have high regard for the quality of work produced by Deep Research.
- Tyler Cowen describes Deep Research as a top bargain in technology, highlighting its speed and efficiency.
- Deep Research is perceived to handle a single-digit percentage of all economically valuable tasks, indicating a significant milestone in its capabilities.
- Users have consistently praised the efficiency and value of Deep Research, suggesting it meets and often exceeds expectations in academic and professional settings.
- Additional testimonials highlight its role in streamlining research processes, saving time, and reducing the workload for researchers and professionals.
4. ποΈ Interview with Gemini Deep Research Creators
4.1. Emergence of AI Clones and Market Competition
4.2. Introduction to Gemini Deep Research
5. π Understanding Deep Research Functionality
5.1. π§ Model Fine-Tuning and Evaluations
5.2. π€ AI Engineer Summit Announcement
6. π§© Deep Research: Challenges and Solutions
- Deep Research, a feature of Gemini, acts as a personal research assistant to help users learn about topics deeply and quickly.
- It supports users in rapidly gaining an understanding of new subjects, facilitating a progression from zero to 50 in knowledge efficiently.
- The feature operates by taking a user's query, conducting a web browse for approximately five minutes, and generating a detailed research report for user review.
- Users can further engage by asking follow-up questions based on the generated research report.
- Deep Research is particularly effective for handling broad queries where users need to quickly acquire foundational knowledge.
- An example scenario might involve a user needing to understand the basics of quantum computing; in this case, Deep Research would provide a comprehensive overview and allow for further question-driven exploration.
7. π‘ Future of AI and Deep Research
7.1. Deep Research Challenges
7.2. Strategies for Effective Deep Research
8. π€ AI Engineer Summit and Industry Insights
- AI models should engage in second-order thinking and test ideas in environments providing feedback for continuous learning, enhancing adaptability and strategic thinking.
- Code sandboxes are effective for end-to-end testing but lack coverage in complex domains like physics engines, highlighting the need for domain-specific testing environments.
- Personalization is key, with AI systems needing to tailor content delivery based on user backgrounds, such as varying educational levels, to enhance engagement and relevance.
- Multimodal reports, incorporating text, charts, maps, and images, are essential for interactive and engaging AI outputs, improving user comprehension and interaction.
- AI development should focus on core functionalities with excellence, allowing for modular expansion and standardization of common capabilities across platforms.
- Deep research should prioritize personalization and memory, enabling AI to remember user interactions and preferences, thereby enhancing user experience.
- Security and access control are crucial as much valuable data is protected by authentication walls. Effective credential management protocols are necessary for secure AI access to user-specific data.
- The rapid evolution of AI tools requires adaptable strategies to prevent obsolescence, emphasizing forward-thinking approaches in AI development.
- Conferences and summits are critical for sharing insights and developing industry protocols, fostering collaboration and innovation in the fast-evolving AI landscape.
- There is a distinct difference in focus between consumer-facing and B2B AI applications, reflecting diverse industry needs and opportunities.
- Demand for deep research capabilities tailored to specific organizational needs is growing, particularly in accessing and analyzing internal company documents.
Included Channels
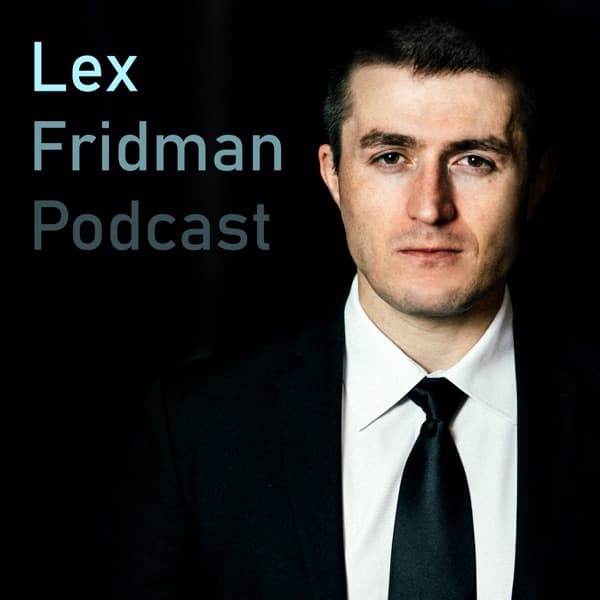
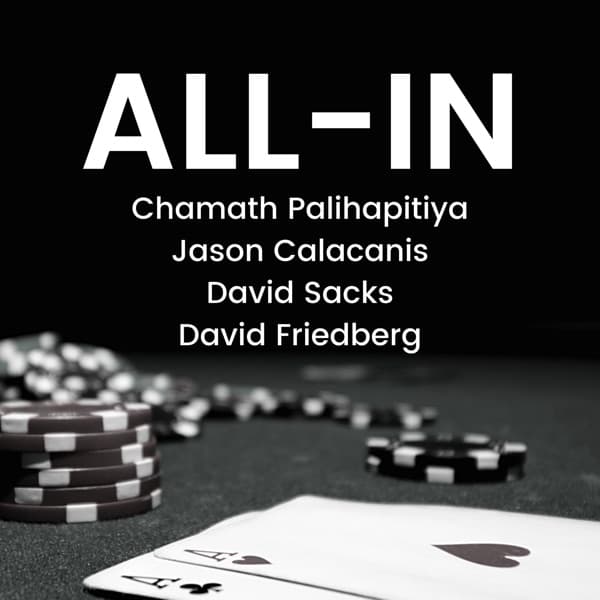
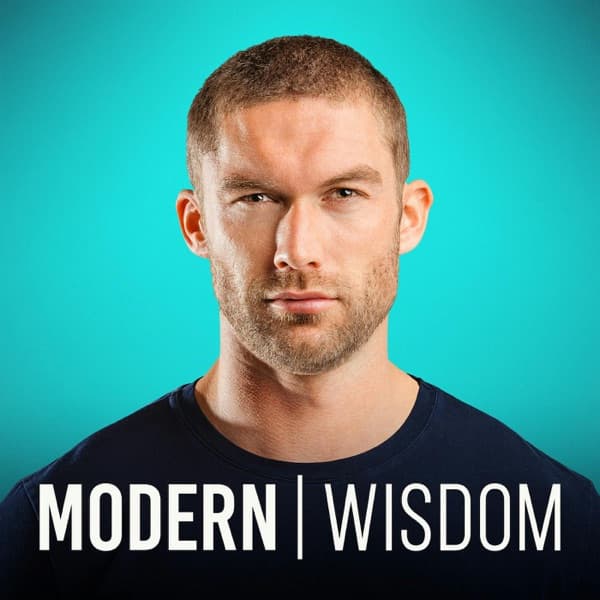
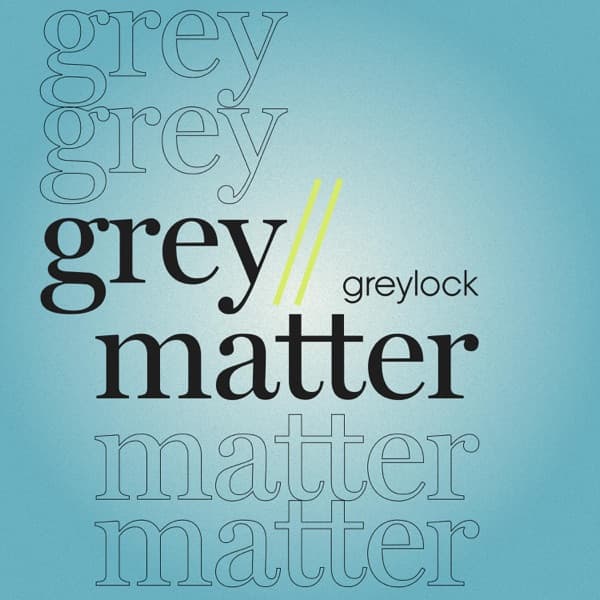
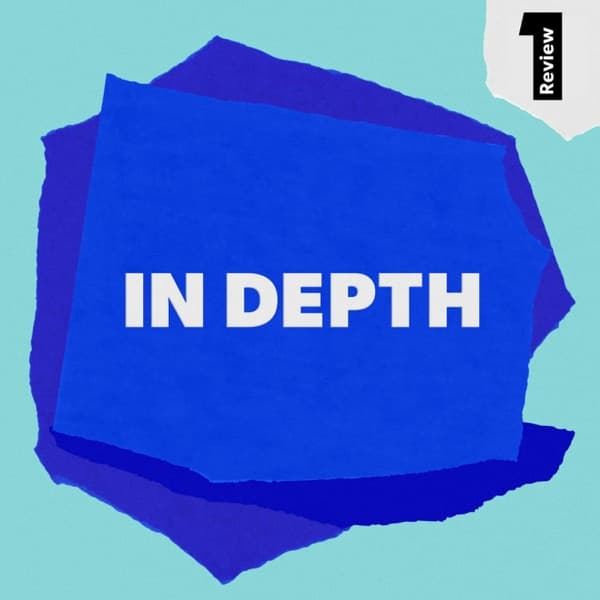
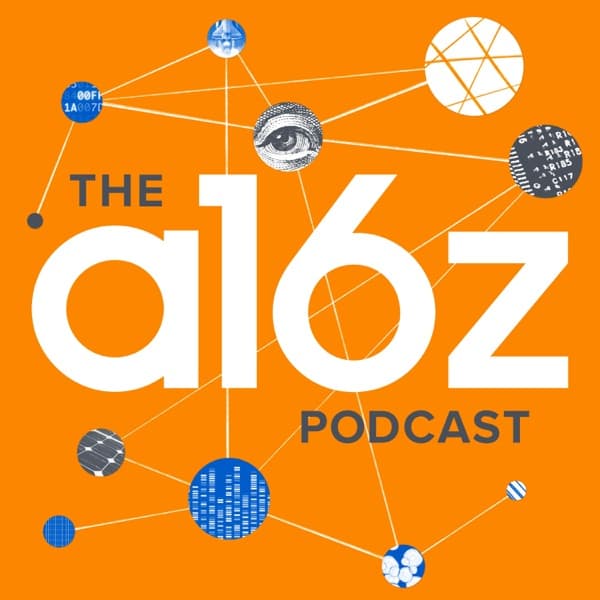
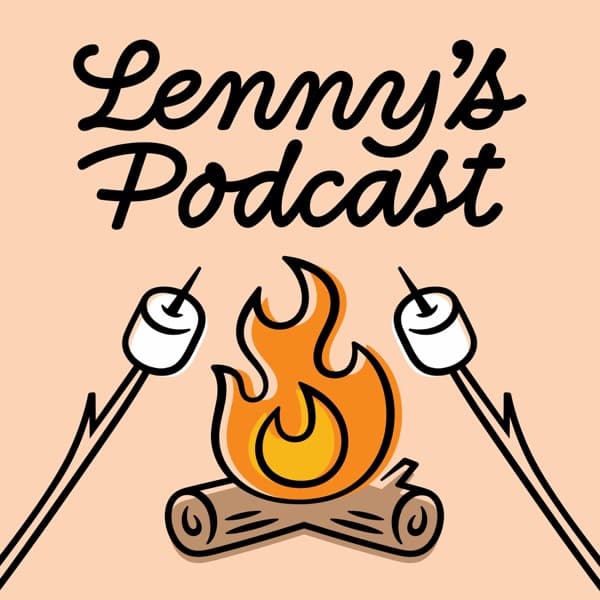
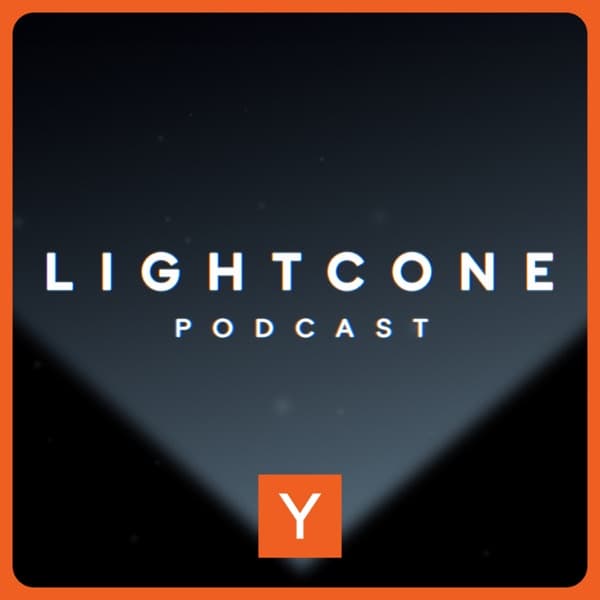
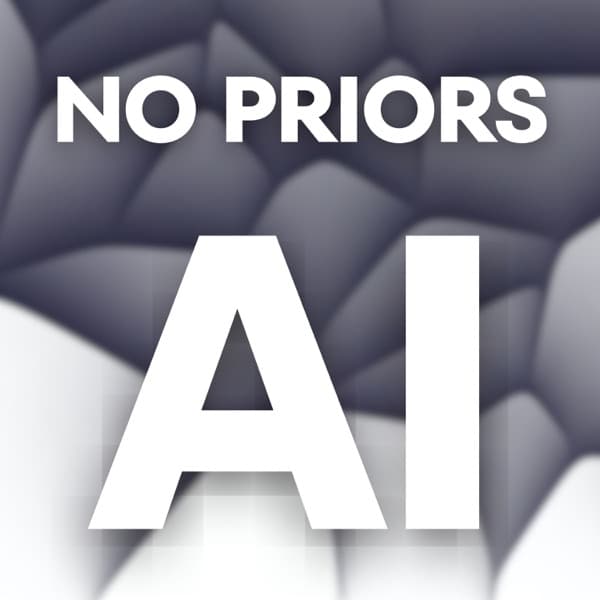
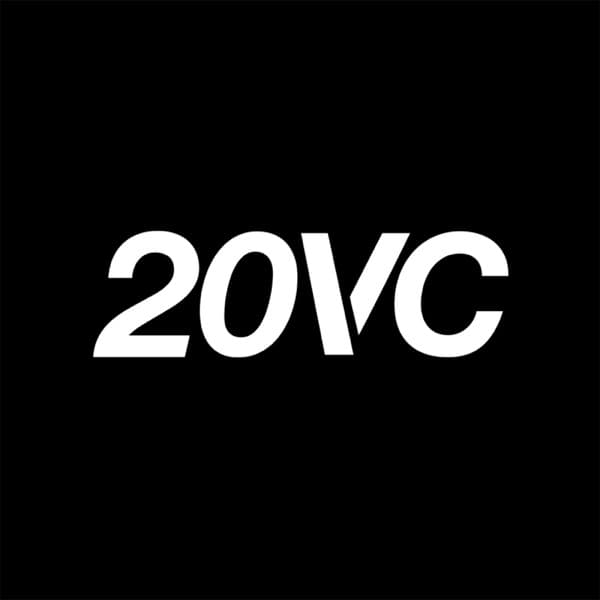
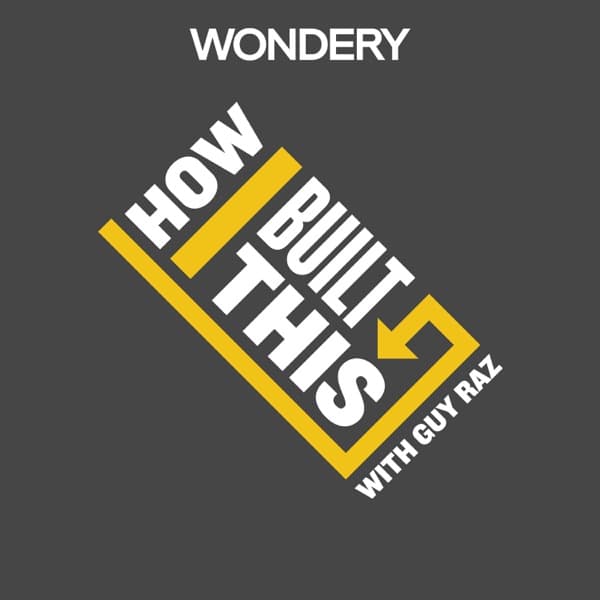
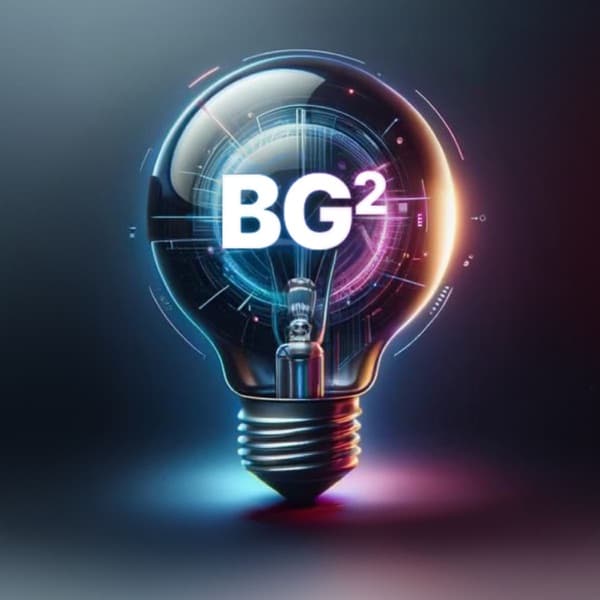
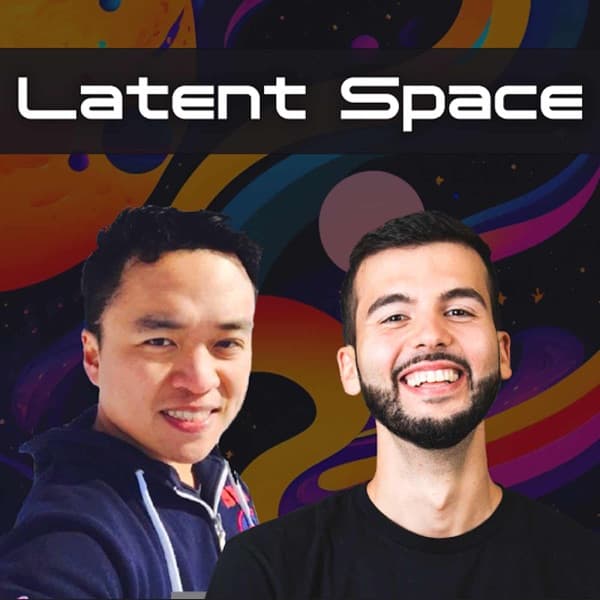