Latent Space: The AI Engineer Podcast: The video discusses advancements and challenges in AI agents, focusing on their applications, development, and future potential.
a16z Podcast: The video discusses the evolving landscape of AI, focusing on the impact of generative AI models on search engines and the strategic importance of AI infrastructure for nations.
Latent Space: The AI Engineer Podcast - 2024 in Agents [LS Live! @ NeurIPS 2024]
![2024 in Agents [LS Live! @ NeurIPS 2024]](/_next/image?url=https%3A%2F%2Fis1-ssl.mzstatic.com%2Fimage%2Fthumb%2FPodcasts116%2Fv4%2F97%2F39%2Fd6%2F9739d6fc-d55e-483b-c8fd-66764254ab1d%2Fmza_11445832196745209483.jpg%2F600x600bb.jpg&w=1920&q=75)
The video is a keynote from a mini-conference at NeurIPS 2024, highlighting the progress and challenges in AI agents. Professor Graham Newbig discusses the state of large language model (LLM) agents, emphasizing their increasing role in various domains. He highlights the success of Open Hands, a startup focused on open-source coding agents, and discusses the practical applications of agents in tasks like web browsing, coding, and software development. Newbig shares insights into the tools and methodologies used to enhance agent capabilities, such as program execution, file editing, and web browsing. He also addresses the importance of human-agent interfaces and the need for agents to have good instruction-following abilities, error recovery, and environment understanding. The video also covers the evaluation of agents, the importance of planning, and the potential for self-improving agents. Newbig predicts that by 2025, large language models will be more focused on agentic applications, leading to increased competition and reduced costs. He emphasizes the need for accessible and affordable AI tools, advocating for open-source solutions to democratize AI technology.
Key Points:
- AI agents are increasingly used in web browsing, coding, and software development, with tools like Open Hands leading the way.
- Effective agent design requires good instruction-following, error recovery, and environment understanding.
- Human-agent interfaces are crucial for smooth interaction, requiring clear communication and feedback mechanisms.
- Future AI models will focus more on agentic applications, increasing competition and reducing costs.
- Open-source solutions are vital for making AI tools accessible and affordable, promoting democratization of technology.
Details:
1. 🎉 Welcome to Latent Space Live
- Latent Space Live is a mini-conference held at NeurIPS 2024 in Vancouver.
- The event marks the first occurrence of Latent Space Live.
- Charlie, an AI, serves as the co-host for the event.
- The session includes a recap of the best moments from the conference.
2. 📊 Survey Insights and Speaker Highlights
- A comprehensive survey was conducted with over 900 participants to identify key topics of interest.
- The survey included questions on preferred topics, speaker preferences, and desired outcomes from the event.
- Data from the survey was analyzed to determine the most requested topics and areas of interest.
- Speakers were selected from the Latent Space Network, ensuring they matched the topics identified as high priority by participants.
- The selection process focused on expertise, relevance to the identified topics, and speaker engagement capabilities.
3. 👥 Audience Engagement
- The event successfully engaged 200 attendees in person, while over 2,200 viewers participated through live online streaming, highlighting a strong hybrid engagement strategy.
4. 🔍 Keynote on LLM Agents
- The keynote introduces the current state of LLM agents, highlighting recent advancements and applications.
- Professor Graham Newbig, a leading expert in the field, returns to discuss these developments.
- Recent advancements include improved natural language understanding and generation capabilities, enabling more sophisticated applications in various industries.
- Applications of LLM agents now extend to personalized customer service, automated content creation, and advanced data analysis.
5. 🚀 Achievements in AI and Agents
- All Hands, formerly known as Open Hands, has achieved significant success this year, ranking number one on the hardest SWE bench full leaderboard with a 29% score.
- They are also performing well on the smaller SWE bench verified, holding a 53% score, although trailing behind Amazon Q Devlo and OpenAI's O3 results at 71.7%.
- The SWE bench full leaderboard and SWE bench verified are benchmarks used to evaluate the performance and capabilities of AI systems, highlighting the competitive landscape in AI development.
6. 🤖 The Rise of AI Agents in 2025
- 2025 is anticipated to be the year of AI agents, with major players like OpenAI, DeepMind, and Anthropic focusing on consumer encoding agents, vision-based computer-using agents, and multi-agent systems.
- Significant progress has been made in the practical reliability and applications of AI agents across various domains.
- Cognition AI's Devon had a major launch this year, indicating strong advancements in AI agent technology.
- Cursor Composer and Codium's Windsurf Cascade have become notable successes in the IDE Arena, showcasing the growing impact of AI agents.
- StackBlitz's Bolt, Lovable, and Vercel's V0 have experienced explosive revenue growth, highlighting the financial potential of AI agents.
- Customer support agent Sierra has reached a valuation of $4 billion, demonstrating the economic value of AI in customer service.
- Search agent Perplexity is now valued at $9 billion, underscoring the significant market impact of AI-driven search solutions.
7. 📚 Notable Papers and Challenges in AI
- The focus was on understanding the most notable papers of the year in AI agents, which provided insights into the latest advancements and methodologies.
- Graham highlighted eight perennial problems in building AI agents, which include issues like scalability, adaptability, and ethical considerations.
- These challenges are critical for the development of more robust and effective AI systems.
- The discussion emphasized the importance of addressing these challenges to push the boundaries of what AI agents can achieve.
8. 🎓 Professor Graham's Insights
8.1. Popularity and Engagement
8.2. Curated List of Best Papers
8.3. Accessibility via YouTube
9. 🧠 Exploring AI Agents in 2024
- In 2024, the landscape of AI agents is vast and diverse, offering a wide range of capabilities and applications.
- The speaker shares insights based on personal experience, emphasizing the importance of practical tools in enhancing productivity.
- Key tools such as web browsers, terminals, file systems, and text/code editors are highlighted as essential for accomplishing 99% of daily tasks.
- These tools are equated to a powerful toolkit that significantly boosts productivity and problem-solving abilities.
- The discussion suggests that AI agents equipped with these tools can handle complex tasks efficiently, reflecting their strategic importance in modern workflows.
10. 🛠️ Tools and Capabilities of AI Agents
- AI agents are being developed to effectively manipulate tasks such as web browsing and coding, showcasing their potential in automating complex processes.
- The speaker, a professor at CMU and chief scientist at All Hands AI, is actively involved in creating open-source coding agents, emphasizing the importance of community-driven development.
- Open Hands is an open-source coding agent framework maintained by the speaker, providing a platform for collaborative innovation in AI-driven coding solutions.
- The speaker utilizes coding agents 5 to 10 times daily, demonstrating their practical utility in solving coding tasks efficiently.
- Live demonstrations of coding agents can be challenging and may not always work as expected, highlighting the need for continuous improvement and testing.
11. 💻 Live Demo: Coding Agents in Action
11.1. Creating Scatter Plots for SWE Bench Score
11.2. Switching to Resend.com for Email Services
11.3. Enhancing Software for Agent Contribution Monitoring
12. 🔍 Analyzing AI Agent Performance
12.1. Performance on SWEBench Normal Dataset
12.2. Performance on SWEBench Verified Dataset
13. 🤔 Designing Effective AI Agents
- In 2024, methodologies for designing AI agents have significantly improved, focusing on complex tasks like website navigation and code writing.
- A critical aspect is the agent-computer interface, ensuring agents can interact effectively with computers and have the necessary tools.
- Agents are provided with granular tools and APIs for specific tasks, such as determining the most cost-effective country to purchase a smartphone, which may require around 30 tool calls.
- OpenHands allows coding agents to call arbitrary Python code, enabling multiple tool calls simultaneously, reducing the need for numerous individual LLM calls.
- This approach enhances efficiency by allowing error correction and simplifying complex task execution.
- The tools required by an agent depend on the specific use case, emphasizing the importance of tailoring tools to the task.
14. 👥 Human-Agent Interaction
14.1. Tool Capabilities
14.2. Advanced Functionalities
15. 🧩 Choosing the Right Language Model
- The human-agent interface is crucial for interaction, yet remains a challenging area without definitive solutions. Effective design principles include presenting information in an understandable format, such as English descriptions of agent actions, to enhance user comprehension.
- Users can explore detailed actions by accessing additional resources like Jupyter notebooks, which enhances transparency and control over AI processes.
- Integration into existing user environments is prioritized, with tools like chat UIs and GitHub plugins facilitating seamless interaction and practical application of AI.
- The OpenHands agent exemplifies practical AI application by autonomously resolving issues, such as fixing tests on GitHub, showcasing its utility in real-world scenarios.
- Remote runtime capabilities allow for launching multiple agents to tackle various problems simultaneously, offering scalability and efficiency.
- Interface design and functionality should be tailored to specific use cases, such as coding or insurance auditing, to maximize effectiveness and user satisfaction.
16. 🔍 Planning and Workflow in AI
- Selecting a language model with strong instruction-following ability is crucial for agentic LMs, enabling applications like automated customer service and data analysis.
- Effective tool use and coding abilities are essential for language models to perform tasks efficiently, such as automating repetitive coding tasks or integrating with APIs.
- Understanding the environment, such as web pages, is necessary for building web agents, requiring either vision or text comprehension, which can be applied in web scraping or online data monitoring.
- Error awareness and recovery ability are critical for language models to identify mistakes and develop alternative strategies, improving reliability in dynamic environments.
- Claude demonstrates superior capabilities in instruction following, error recovery, and adaptability compared to other models like GPT-4-0, making it suitable for complex problem-solving tasks.
- In evaluations, Claude outperformed other models, including GPT-40-01-mini LAMA-405b and DeepSeq 2.5, as a code agent, highlighting its efficiency in executing code-based tasks.
- Despite being a few months old, evaluations suggest Claude remains the leading model, with other closed models and open models lagging behind, indicating its advanced development and potential for future applications.
17. 🔍 Exploration and Search in AI
- Implementing a structured workflow for solving GitHub issues can enhance efficiency. The recommended workflow includes reproducing the issue, writing tests to demonstrate it, running tests to ensure failure, fixing the issue, and rerunning tests to confirm resolution.
- Using language models to generate plans on the fly is a valid alternative to curated plans, offering flexibility in problem-solving.
- Explicit structure through multi-agent systems can be beneficial but may limit flexibility when deviations from the plan are necessary. Single-agent systems with strong instruction-following capabilities can offer more adaptability.
- The Coact paper highlights the importance of being able to deviate from plans when necessary, emphasizing the need for flexibility in AI-driven workflows.
- Specifying common workflows in software development, such as fixing GitHub actions, can streamline repetitive tasks and improve efficiency.
- Research on manual prompting and agent workflow memory shows promise in creating self-improving agents that learn from past successes, enhancing future task performance.
18. 📊 Evaluation and Benchmarks
18.1. Self-Learning and Improvement
18.2. Exploration in Coding
18.3. Exploration in Web Agents
18.4. Search Path Strategies
18.5. Evaluation and Benchmarks
19. 🔮 Predictions for AI Agents
- By mid-2025, every large language model (LLM) trainer will focus on training models as agents, leading to better agent models.
- Competition among LLMs will increase, driving prices down and making smaller models competitive as agents.
- Instruction-following abilities in agentic contexts will improve, reducing the need for manual engineering of workflows.
- Error correction abilities will enhance, decreasing the likelihood of models getting stuck in loops.
- Agent benchmarks like WebArena and SweeBench will become too easy, prompting the development of more challenging benchmarks.
- Current success rates on SweeBench are around 53-55%, but actual model abilities may be closer to 30-40%.
- The challenge will be to make human-agent interactions smoother, allowing humans to audit agent work effectively.
- The integration of programming agents into other industries will be crucial, with programmers likely adopting them naturally.
20. 🌍 Making AI Accessible
20.1. API Usage and Interaction Accuracy
20.2. Open Source and Accessibility
21. 🤝 Call to Action for Open Source
- Duolingo's model of charging U.S. users $20/month to provide free language education in South America is an example of making services affordable and accessible.
- Encouragement to contribute to open source projects, such as Open Hands, and to use, research, and train strong open source models.
- Call to action for training models for coding agents to make them affordable.
- Individuals can contribute by participating in open source projects, sharing knowledge, and supporting the development of accessible technology.
22. ❓ Q&A Session: AI Agent Challenges
22.1. AI Agent Performance Metrics
22.2. Challenges Faced by AI Agents
23. 🤔 Addressing AI Agent Limitations
- AI agents interact with websites using three main methods: (1) Clicking on specific pixel values from screenshots, which is unreliable due to misclicks; (2) Parsing HTML to identify elements, often condensed into an accessibility tree for efficiency; (3) A hybrid approach using screenshots and textual summaries, considered the most effective for accurate interactions.
- Current AI agents primarily rely on text for web interactions, with plans to integrate visual elements. Two modalities for web browsing have been implemented: full interaction with website elements and a Markdown conversion for concise information gathering.
- Microagents enhance task-specific interactions by being triggered through keywords like 'GitHub' or 'NPM', providing tailored instructions to avoid common pitfalls such as interactive terminal stalls.
- The Anthropic Model Context Protocol (MCP) is seen as redundant for some existing APIs like GitHub, as it duplicates functionality without significant added value.
- AI agents currently solve about 30% of issues initially. Failures often result from insufficient information gathering before task execution. Improved prompting and scaffolding can enhance performance, as shown by a debugging example where the agent successfully identified potential causes for a bug.
24. 🔄 Self-Improving AI Agents
- A strong language model with infinite context is a foundational approach for self-improving agents, enabling learning from past experiences, though it is costly and complex to implement.
- Current methods like RAG (Retrieval-Augmented Generation) are not very effective for indexing into infinite context, highlighting the need for more efficient solutions.
- Fine-tuning involves adding successful examples to the model, enhancing its performance by learning from past successes.
- Building a skill library, akin to Voyager's approach, allows for the accumulation of skills over time, improving the agent's efficiency and capability.
- Workflows or playbooks can be used to document and persist successful processes, creating a skill library that serves as a shortcut for previously failed attempts.
- GitHub fine-grained authentication tokens are recommended for managing agent authentication, providing granular permission control and enhancing security.
- The lack of fine-grained authentication in most APIs suggests a need for better preparation for agent integration.
- Practical examples include deploying scripts and managing pull requests, illustrating the iterative nature of development and the importance of continuous improvement.
a16z Podcast - The Race for AI—Search, National Infrastructure, & On-Device AI
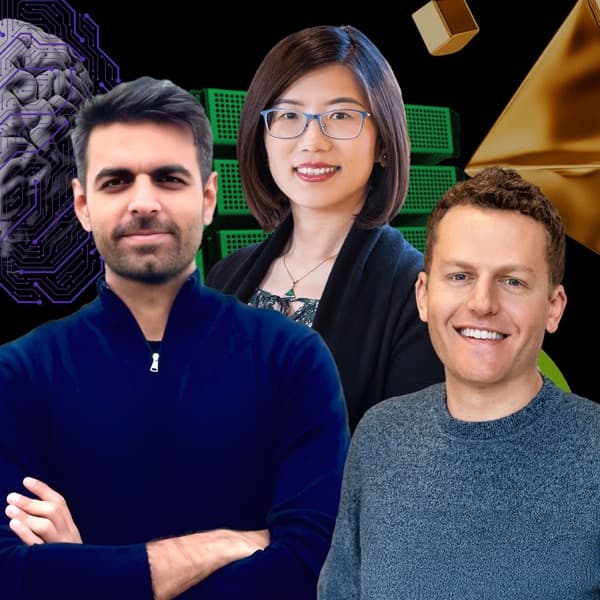
The discussion highlights the transformative impact of AI on search engines, with generative AI models like ChatGPT and Perplexity challenging Google's dominance. These models offer longer, more conversational queries and are gaining traction due to their ability to provide synthesized answers without cluttered ads. The video also explores the strategic importance of AI infrastructure for nations, emphasizing the need for countries to decide whether to build or buy AI capabilities. Smaller nations are encouraged to form alliances with AI 'hypercenters' to leverage their strengths and compensate for local limitations. The conversation touches on the potential for on-device AI models to enhance user experiences by providing real-time, privacy-focused applications, which could reshape consumer interactions with technology.
Key Points:
- Generative AI models are disrupting traditional search engines by offering more detailed and conversational queries.
- AI infrastructure is becoming a critical national asset, with countries needing to decide whether to build or buy capabilities.
- On-device AI models are expected to grow, offering real-time and privacy-focused applications.
- Smaller nations should consider alliances with AI 'hypercenters' to enhance their AI capabilities.
- The shift towards AI-driven search and infrastructure presents both opportunities and challenges for global tech dynamics.
Details:
1. 📜 AI Legislation Surge
- In 2024, over 700 pieces of state-level legislation were AI-specific, indicating a significant increase in regulatory focus on AI technologies.
- The surge in AI legislation reflects growing concerns over ethical use, privacy, and security in AI applications.
- Examples of legislation include laws mandating transparency in AI algorithms and regulations on AI use in surveillance.
- This legislative trend is driven by the rapid adoption of AI across various sectors, necessitating updated legal frameworks to address new challenges.
- The implications of these laws include increased compliance requirements for AI developers and potential impacts on innovation.
2. 🔍 Search Query Comparison
- The average query on Perplexity is 10 to 11 words, indicating users tend to input more detailed queries, possibly due to the platform's design encouraging comprehensive questions.
- The average search on Google is 2 to 3 keywords, suggesting users prefer concise queries, likely due to Google's efficiency in handling short, targeted searches.
- Longer queries on Perplexity may lead to more precise results, enhancing user satisfaction by addressing specific information needs.
- The concise nature of Google searches reflects its ability to quickly deliver relevant results, catering to users seeking fast answers.
3. 💼 Government Investments & Founders' Impact
3.1. Government Purchases
3.2. AR Experience Reimagined
3.3. Founders' Generational Impact
4. 📅 Reflecting on Technological Milestones
- Wikipedia has been around for 24 years, highlighting its long-standing presence and influence in the digital information space.
- The iPhone has been on the market for 18 years, marking nearly two decades of impact on mobile technology and consumer behavior.
- It has been 16 years since the release of the Bitcoin white paper, indicating the significant time cryptocurrency has had to evolve and influence financial systems.
- Social media platforms like Facebook have been influential for over 19 years, reshaping communication and social interaction globally.
- Artificial Intelligence advancements have accelerated over the past decade, significantly impacting industries such as healthcare, finance, and transportation.
5. 🌊 A16Z's Big Ideas for 2025
- A16Z is accelerating innovation as we approach 2025, collaborating with partners to shape the future.
- Key predictions include a new age of maritime exploration, leveraging technology for deeper oceanic understanding.
- Advancements in programming medicine are expected to revolutionize healthcare, making treatments more precise and personalized.
- AI is anticipated to introduce continuous schemes, transforming industries with ongoing, adaptive processes.
- A focus on democratizing access to miracle drugs aims to make life-saving treatments more widely available and affordable.
6. 🔧 Bridging Hardware-Software Gap
- Focus on closing the hardware-software chasm to enhance integration and performance by aligning technological capabilities with business needs.
- Utilize game technology to drive innovation and efficiency in future business operations, leveraging its interactive and immersive capabilities to improve user engagement and training.
- Implement super staffing strategies in healthcare to optimize workforce management and improve patient care, ensuring that staffing levels are aligned with patient needs and operational demands.
7. 🎮 Exploring Diverse Tech Sectors
- The series covers diverse sectors including American Dynamism, healthcare, fintech, and games, highlighting their potential for innovation and growth.
- Listeners are encouraged to explore a comprehensive list of 50 big ideas available at a16z.com/bigideas, providing a strategic resource for understanding emerging trends.
- Part one of the series focuses on a specific topic, urging listeners to catch up on missed content to gain a full understanding of the discussed sectors.
8. ⚠️ Legal and Investment Disclaimers
- The content is for informational purposes only and should not be taken as legal, business, tax, or investment advice.
- It should not be used to evaluate any investment or security.
- The information is not directed at any investors or potential investors in any A16Z fund.
9. 🤖 AI's Growing Influence
- Artificial intelligence is the central topic of discussion for the year, indicating its significant impact across various sectors.
- Major companies like Google are in a competitive race to innovate and integrate AI, moving beyond traditional search methods.
- Sovereign countries are actively seeking to leverage AI as a strategic advantage in the global landscape.
- Device companies are exploring their roles in the evolving AI ecosystem, particularly as AI technology advances towards edge computing.
10. 🔍 The Future of Search Engines
- The search monopoly is predicted to end in 2025, with Google's control over 90% of U.S. search slipping.
- A recent U.S. antitrust ruling is encouraging Apple and other phone manufacturers to empower alternative search providers.
- Generative AI is impacting search, with ChatGBT having 250 million weekly active users.
- Answer Engine perplexity is growing 25% month on month, changing search engagement with queries averaging 10 words, three times longer than traditional search.
- Nearly half of these queries lead to follow-up questions, indicating a shift in user behavior.
- 60% of U.S. consumers used a chatbot for research or purchase decisions in the last 30 days.
- Professionals are using domain-specific providers like Causally, ConsenSys, Harvey, and Hebbia for deep work.
- Google's traditional ad and link model is becoming cluttered, leading users to seek more direct answers and depth.
- Google's potential shift to AI results could impact short-term profits.
- The term 'Google' as a verb is under threat, with a race for its replacement underway.
11. ⚖️ Legal Pressures on Google
11.1. Google's Market Dominance and Revenue
11.2. Legal Challenges and Competitive Barriers
12. 🔄 Transition to AI-Powered Search
- Traditional search engines like Google present a long list of links, often with sponsored ads at the top, requiring users to sift through information to find answers.
- AI-powered search tools like ChatGBT, Perplexity, Claude, Character AI, and Poe provide immediate answers, enhancing user experience by reducing the time and effort needed to find information.
- AI-powered search tools process queries using natural language processing and machine learning algorithms, allowing them to understand context and deliver more precise answers.
- In scenarios where users need quick, specific information, AI-powered search tools outperform traditional search engines by eliminating the need to navigate through multiple links.
13. 📈 Rise of AI Search Engines
13.1. Popularity and Adoption of AI Search Engines
13.2. Features and User Experience of AI Search Engines
14. 🌐 Search Market Dynamics
14.1. AI-Driven Tools and Consumer Behavior
14.2. Emergence of Verticalized Search Engines
14.3. Market Dominance and Network Effects
15. 🌍 AI Infrastructure and Global Dynamics
15.1. Vertical vs. Horizontal Software
15.2. Consolidation in Search Engines
15.3. Enterprise and Consumer Search Blending
15.4. Ad-Based vs. Subscription Models
15.5. Future Opportunities and Challenges
16. 🏗️ Building AI Infrastructure Independence
- AI is a transformative general-purpose technology, akin to electricity or the printing press, with extensive applications and significant economic impact.
- Countries must decide whether to develop their own AI infrastructure or depend on external providers, a decision that will be pivotal in the next 24 months.
- AI's rapid integration into societies is facilitated by existing digital infrastructure, making it a key factor in national development strategies.
- Smaller nations are advised to form joint ventures with AI 'hypercenters'—countries with the capability to develop and host advanced AI models—to achieve infrastructure independence.
- AI models encode human values based on their training data, which often reflects the cultural norms of the data's origin, emphasizing the need for alignment with national values.
- The economic impact of AI as a general-purpose technology is profound, influencing various sectors and necessitating strategic planning for infrastructure development.
- Examples of smaller nations successfully partnering with AI hypercenters include collaborations that enhance technological capabilities and foster economic growth.
17. 🌐 Global AI Alliances and Values
17.1. Aligning Value Systems
17.2. Historical Precedent of Currency
17.3. AI Hypercenters, Compute Deserts, and Resource Value
17.4. Key Ingredients for AI Leadership
17.5. Comparative Advantage and Alliances
17.6. Collaborative AI Models
18. 🔧 Sovereignty in AI Infrastructure
- Countries and large companies need to assess which parts of the AI infrastructure stack are critical for independence based on their existing assets and strengths.
- Building AI infrastructure, especially at the chip and lithography layers, can take years or even decades, as exemplified by ASML, a Dutch company that produces essential lithography machines costing $200 million each, with $23 billion in revenue, 40% from China.
- ASML is the only company capable of producing EUV lithography machines, highlighting the challenge for countries like the US to replicate such capabilities quickly.
- Smaller countries might find it more feasible to train local AI models if they have leading research teams, although only a few teams globally have this capability.
- Sovereign AI infrastructure doesn't require 100% ownership of every stack component but rather independence from critical parts controlled by untrusted entities.
- For instance, Taiwan has successfully developed a robust semiconductor industry, demonstrating that strategic focus on specific layers of the AI stack can lead to sovereignty.
- Countries should prioritize developing capabilities in areas where they can leverage existing strengths, such as software development or AI research, to achieve partial sovereignty.
19. 🏢 Private vs. Government Roles in AI
- In China, the PRC 2017 National Intelligence Law mandates that Chinese companies must make their technology available to the government, highlighting a clear line between government and private enterprise.
- In contrast, the United States and allied countries generally protect private sector technology from mandatory government access, except for specific cases like dual-use or defense-funded technologies.
- The G5 countries (US, Canada, UK, Australia, New Zealand) have a joint framework for categorizing infrastructure, but AI models are not typically classified as dual-use or under national security protection.
- The historical trend in technology development suggests that minimizing bureaucratic barriers and leveraging the best talents leads to success.
20. 🇺🇸 U.S. AI Strategy and Challenges
- The U.S. private market is effectively responding to market demand in the compute sector, with major infrastructure businesses being chip and computing companies.
- Data regulation in the U.S. is fragmented, with over 700 pieces of state-level AI-specific legislation in 2024, leading to a patchwork of approaches.
- The lack of a unified federal framework for data regulation, especially for AI training, is a significant handicap for the U.S., causing compliance confusion among companies.
- In contrast, countries with less stringent copyright and IP laws are advancing more rapidly in AI development.
- U.S. companies are hindered by unclear and inconsistent state regulations, which are often impossible to adhere to, unlike a potential unified federal approach.
21. 🔋 Energy and Compute in AI Development
- The lack of government support for cross-border collaboration is a significant barrier to frontier AI research in the U.S. and allied countries, limiting innovation and development.
- France's strategic decision to embrace nuclear energy two decades ago has resulted in highly efficient data centers, contrasting with the U.S., which has not fully leveraged nuclear energy for AI infrastructure.
- Proposals to hold model developers liable for inference outputs could deter innovation and disproportionately benefit large tech companies, potentially stifling smaller developers.
- Data centers are emerging as critical components of national sovereignty, with countries investing heavily in them as part of their AI supply chain strategies.
- There is an unprecedented demand from governments for NVIDIA's GPUs, with orders being placed 12 to 36 months in advance, indicating the strategic importance of securing compute resources for AI development.
22. 🌟 Visionary Founders in AI
- Founders with deep technical and research backgrounds, often from large hyperscaler labs, are crucial for AI innovation.
- Examples include Arthur Mensch from Mistral and Guillaume Lomp from Meta, who have led significant AI model developments.
- These founders are mission-driven, aiming to solve complex infrastructure problems for large governments.
- Technical founders with academic training are motivated to tackle challenging problems, impacting humanity on a generational scale.
- Arthur Mensch and Guillaume Lomp exemplify the impact of technical expertise in advancing AI capabilities, having developed models that address complex challenges.
23. 📱 On-Device AI and Future Applications
- On-device AI models are expected to become more prevalent due to economic, practical, and privacy considerations.
- Smaller generative AI models will likely dominate in volume and usage, similar to existing machine learning models in apps like Uber and Airbnb.
- Smartphones have significant compute power, comparable to computers from 10-20 years ago, enabling them to run smaller AI models effectively.
- Models with 2 billion to 8 billion parameters can run on devices, providing robust experiences in text, image, and audio generation.
- Diffusion models are smaller and capable, and distillation techniques allow large models to be reduced in size while maintaining capabilities.
- Running AI models on devices improves user experience by reducing latency, enhancing real-time interactions, and maintaining privacy.
- Real-time voice agents and AR experiences are potential applications for on-device AI models.
- Economically, on-device AI could shift costs and efficiencies, though the impact on infrastructure costs is uncertain.
- Hardware developers and model developers are likely to benefit from the proliferation of on-device AI models.
- The trend towards on-device AI is expected to influence the entire supply chain, with significant developments anticipated by 2025.
24. 🔮 Looking Ahead to 2025
- The segment encourages anticipation and preparation for 2025, suggesting that the ideas presented are foundational for future planning.
- Listeners are directed to visit asicsnews.com/bigideas for a comprehensive list of 50 big ideas, indicating a resource for strategic insights and planning.
- The phrase 'It's time to build' implies a call to action, encouraging proactive engagement with the presented ideas.
Included Channels
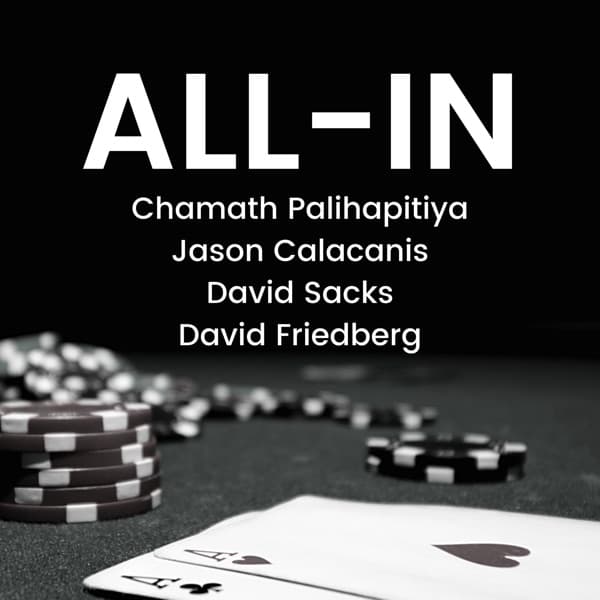
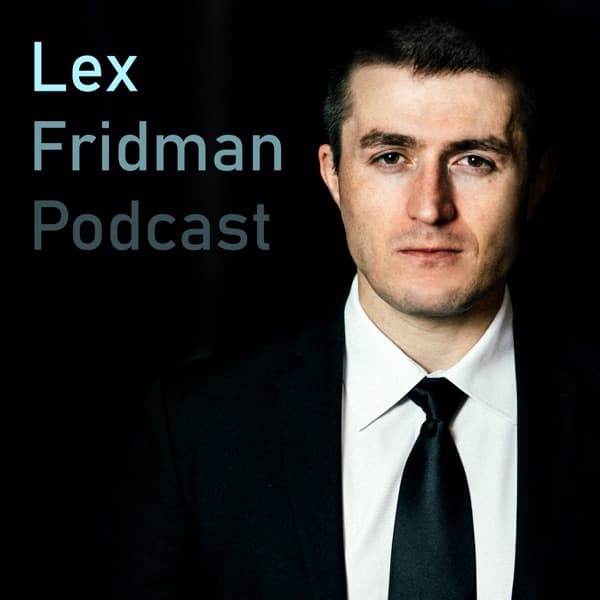
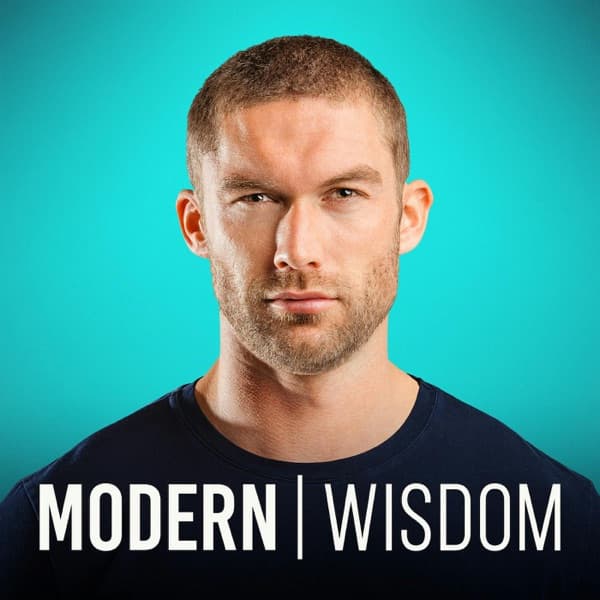
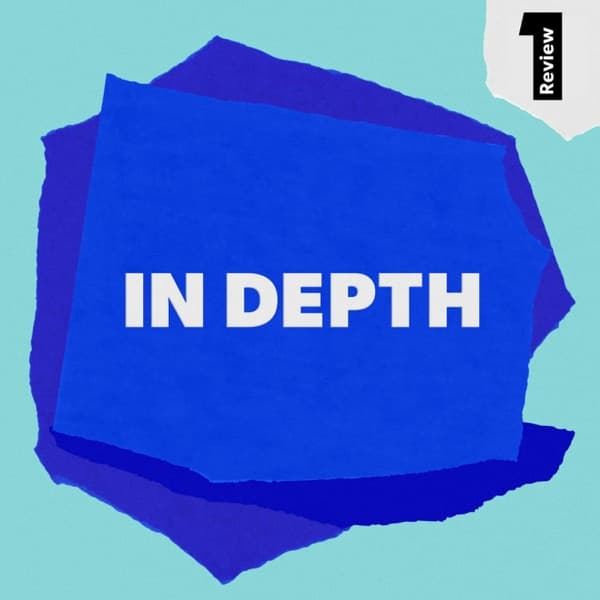
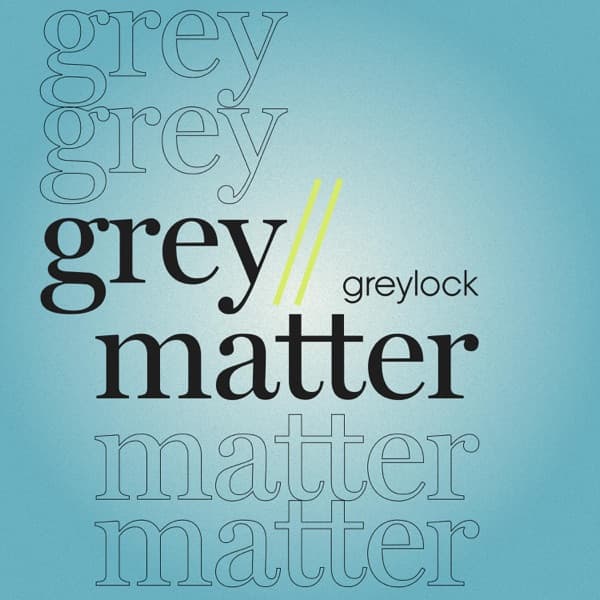
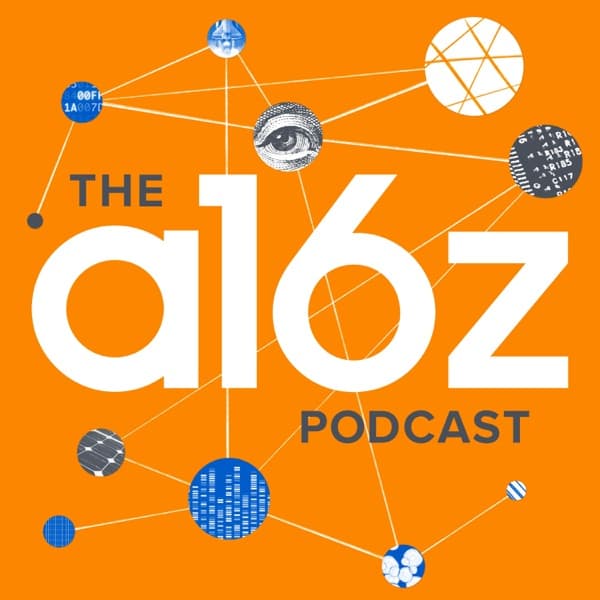
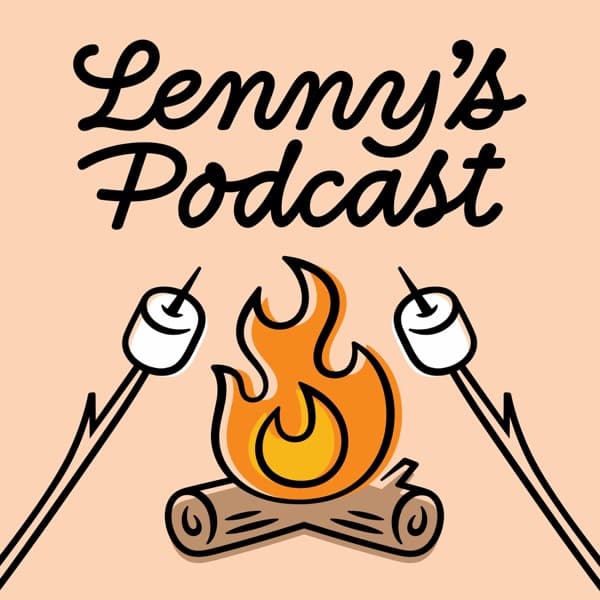
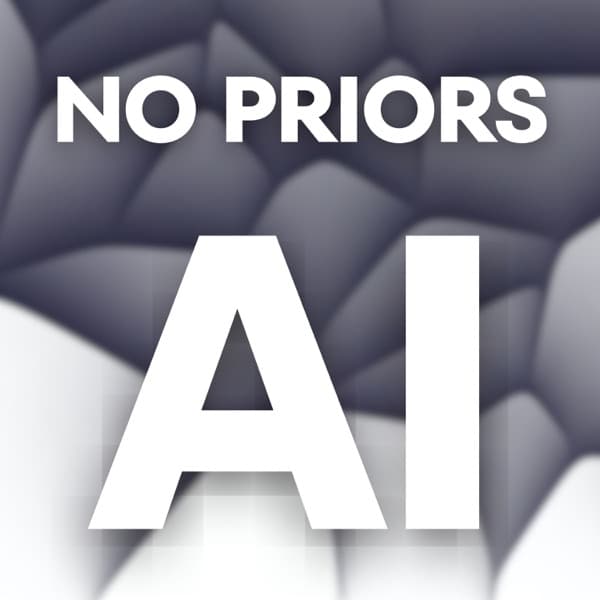
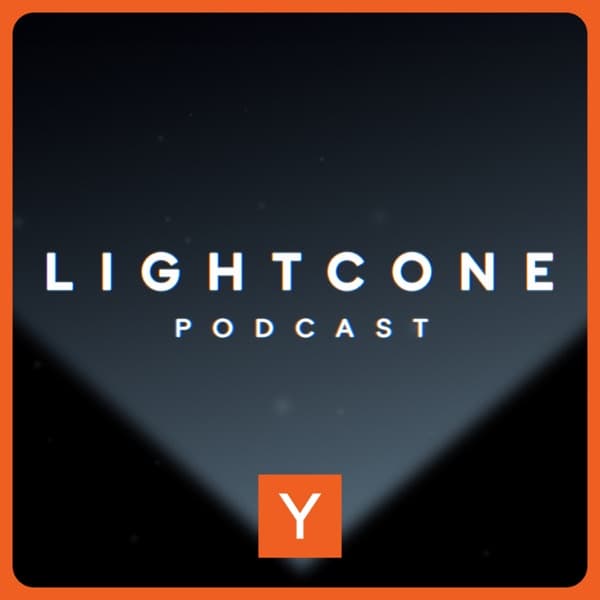
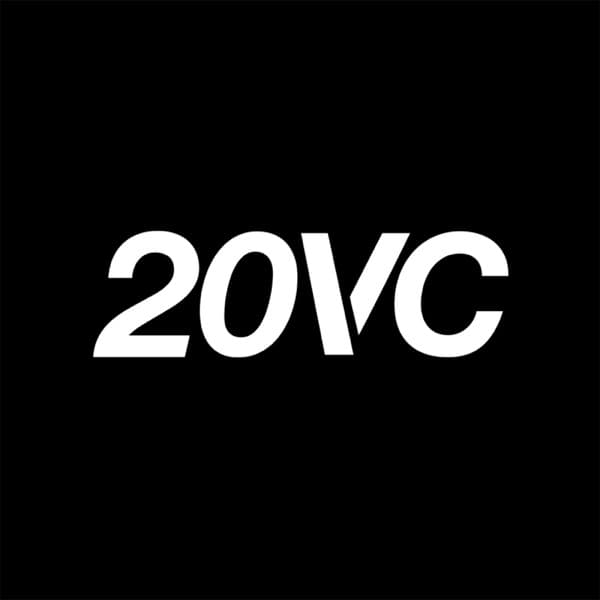
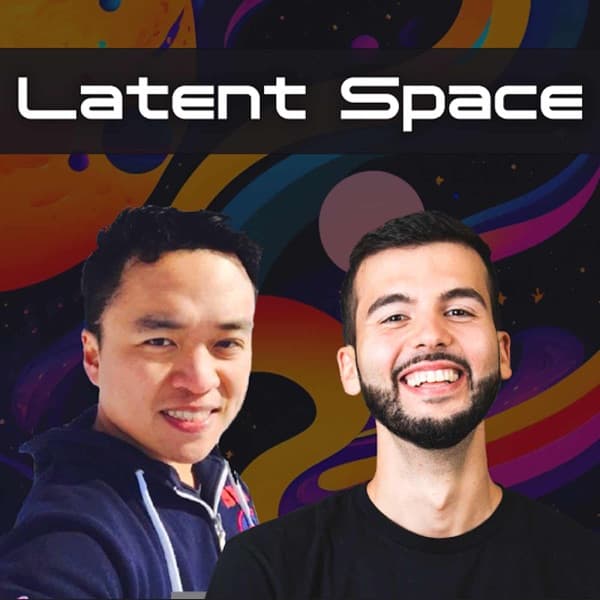
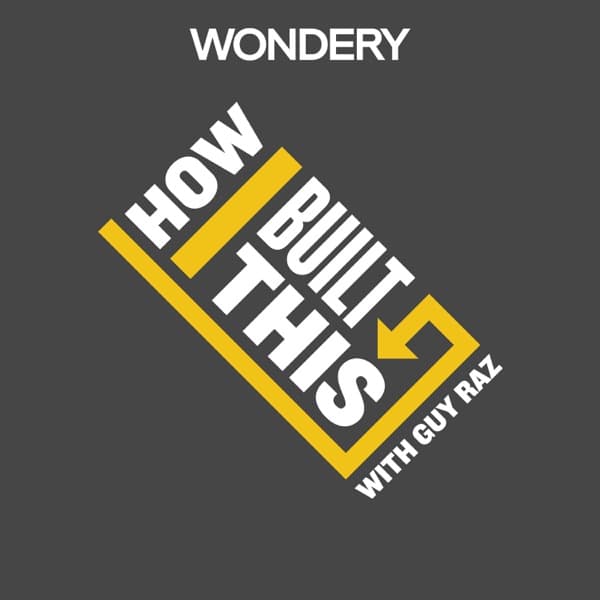
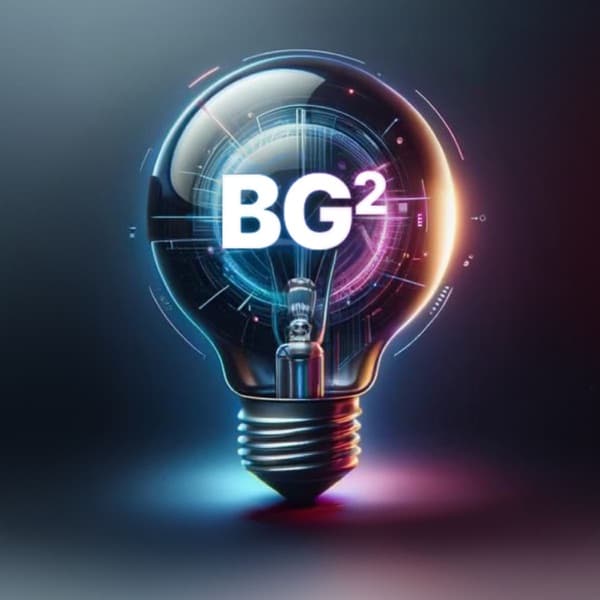